DeepSeek's Impact On American Tech
The paradoxes, the consequences and the details about DeepSeek and its impact
Hi Multis
Everybody’s talking about DeepSeek and its impact on Nvidia (NVDA) and the American tech sector in general. For several days, I studied the details and the impact of DeepSeek.
A few questions I will answer in this article:
Is DeepSeek legit?
Did it use forbidden H100 chips?
How does it disrupt?
Does this mean the end of Nvidia and others?
Did big tech overspend?
Is China the new AI leader now?
What I will do with my Nvidia position.
DeepSeek launched its newest AI iteration, R1, on the day of Trump's inauguration. I don't want to wade into conspiracy theories right away, but I don't rule out that it was not a coincidence. It doesn’t really matter. What is important is the impact the launch had. That impact was deep and profound and that’s what we will discuss in this article.
The Reaction
The R1 launch was a shock to many. The reason is this.
Source: DeepSeek
DeepSeek scores overall (almost) are as good or better than the Western AI models in math, coding, and reasoning. This shocked the world. On top of that, the model was made open source.
Marc Andreessen, who founded Mosaic, the first big web browser and one of the most prominent voices in venture capital now with Andreessen-Horowitz (sometimes referred to as A16Z), posted this on X:
He sent out several posts on X about DeepSeek. This is another one.
And Andreessen was not alone. This is a reaction of Marc Benioff, for example, founder and CEO of Salesforce (CRM), a company with a market cap of $320 billion:
There were also many tweets like this:
Here, you already have it. Deepseek claims to have used only $5.6 million to train the model, making the hyperscalers and OpenAI look ridiculous because all of their spending. There were reactions of disbelief.
To make things worse, DeepSeek is a side project of a quant firm, High-Flyer, not a specialized AI company. High-Flyer is one of the biggest quant hedge funds in China, but relative to American norms, with $8 billion of AUM (assets under management), it's still relatively small. It would be mind-blowing if it was revealed later that High-Flyer also shorted Nvidia and some others. But let’s not think about that now.
Amazingly, High-Flyer's AI arm, DeepSeek, was only started in 2023.
American tech is made ridiculous here, it seems. But is that really true? We'll go into that later in this article.
Are DeepSeek's Claims True?
We can't check the claims of all things DeepSeek writes. With Western companies, you already have to be very careful with the data they provide because, of course, they will always want to look good.
With Chinese companies, it's much worse. While American companies are scrutinized by the SEC (Securities and Exchange Commission) and held to rigorous standards of financial transparency, Chinese companies are not held to the same strict rules.
Moreover, Chinese law prohibits the SEC from investigating Chinese companies, even if they trade on the American market. Take Alibaba (BABA), JD (JD), and Baidu (BIDU), for example. During his previous presidency, Donald Trump threatened to delist all Chinese companies because of this.
But a compromise was reached in which the companies get multiple warnings over multiple years before the delisting. I could see American law becoming stricter again regarding Chinese companies listing in the US under Trump, but that's another discussion.
Let's go back to whether DeepSeek can be believed or not in its technical claim.
ScaleAI CEO Alexandr Wang and Anthropic CEO Dario Amodei both claimed that DeepSeek has 50,000 Nvidia H100 chips. Elon Musk agreed with Wang's assessment, replying "obviously" on X.
(Source)
And, of course, as an American tech CEO, that's a comforting thought. After all, 50,000 H100 GPU chips mean at least $1.5 billion, not merely $5.6 million.
But Ben Thompson of Stratchery disagrees and thinks DeepSeek is legitimate:
Actually, the burden of proof is on the doubters, at least once you understand the V3 architecture.
Remember that bit about DeepSeekMoE: V3 has 671 billion parameters, but only 37 billion parameters in the active expert are computed per token; this equates to 333.3 billion FLOPs of compute per token.
Here I should mention another DeepSeek innovation: while parameters were stored with BF16 or FP32 precision, they were reduced to FP8 precision for calculations; 2048 H800 GPUs have a capacity of 3.97 exoflops, i.e. 3.97 billion billion FLOPS.
The training set, meanwhile, consisted of 14.8 trillion tokens; once you do all of the math it becomes apparent that 2.8 million H800 hours is sufficient for training V3. Again, this was just the final run, not the total cost, but it’s a plausible number.
This reasoning can make sense. I'm not technically developed enough to know who is right.
Thompson thinks that the 50,000 H100 claim from Wang, Amodei and Musk goes back to this tweet:
The H in H100 stands for Hopper, but the H800 is also a Hopper, so this doesn't clarify things that much.
The US export rules do not restrict the H800 import and use in China, while the H100 is officially not allowed to enter China.
So, a Chinese company like DeepSeek will also say older Nvidia chips are used if they used H100 chips in reality. Suppose DeepSeek had H100 chips; how did it get them?
Well, if you look at Nvidia's financials, you see that a big part of Nvidia's revenue still comes from China and Singapore. Of course, Singapore is not China, but bringing GPUs from Singapore to China is easier than bringing them from the US.
But I want to repeat this: we don't know for sure if DeepSeek is hiding the truth here or not. My initial reaction was that it was impossible without H100s but I'm not that sure anymore after my research for this article.
Copying ChatGPT
It's also clear that DeepSeek did something Chinese companies are good at: copying.
Let's go back to DeepSeek. This was still the case a month ago.
(Source)
So, some copying has been done in one way or another. This works through distillation.
Distillation starts with insights from another model by sending inputs to the teacher model, and recording the outputs. Those outputs are then used to train the student model, in this case DeepSeek.
Probably, DeepSeek has done this through OpenAI's API. This clearly violates the terms of service of the models but you can't block it, except if you block a user.
But don't blame DeepSeek and the Chinese for this copying. More and more models have become like GPT -4. OpenAI had a clear lead, but it has mostly disappeared with the release of the different LLM models. So, DeepSeek has probably distilled GPT-4 and could be much cheaper because of that.
It's easy to ridicule OpenAI, Google and Anthropic for investing billions of dollars, but the development costs for new cutting-edge models are just that big. If you can consequently copy most of that work, it's much cheaper, of course. But that doesn't make DeepSeek a simple copycat, mind you.
Why DeepSeek Is Revolutionary
It doesn't even matter if DeepSeek lies about its chips or not. You should not underestimate the accomplishments of Liang Wenfeng and his team.
Liang Wenfeng, source
DeepSeek's R1 is revolutionary. There's no denying that, even if it would be trained on 50,000 smuggled H100 chips.
It uses pure reinforcement learning, and that makes it the first time that the reasoning capabilities of LLMs can be done without supervised fine-tuning (read human intervention).
DeepSeek's first crucial innovation is DeepSeekMoE, which stands for “mixture of experts.” What DeepSeek did was build a sort of "expert team." ChatGPT 3.5 still tried to be everything at once (doctor, coder, teacher, marketer, scientist, etc.).
But just like GPT4, DeepSeek has created specialized experts under one umbrella. They only work when they are called to action, triggered by a prompt. GPT-4 was a MoE model that was believed to have 16 experts with approximately 110 billion parameters each. DeepSeek took this model, but improved it significantly. It made many more and more specialized experts and combined it with shared experts with more generalized knowledge to connect the different experts.
In numbers, ChatGPT 3.5 will activate all 1.8 trillion parameters for every prompt. ChatGPT4 will still activate 110 billion for each expert. DeepSeek has 671B parameters (so only just about a third of ChatGPT) but only 37B are active at once. That means a huge efficiency gain.
DeepSeekMoE made training smarter and faster by changing how it splits up and handles tasks. Normally, these types of models are designed to work quickly when answering questions or solving problems, but they’re slow and complicated to train. DeepSeek found a way to make the training process simpler and less messy, saving time and effort.
There's also DeepSeekMLA, which is probably an even more significant innovation. One of the limitations of LLMs so far is the huge memory requirements for inference, so you and I using ChatGPT or Claude by giving it prompts.
When the model is running, it not only needs to load itself but also everything you’re asking it to consider (called the "context window"). This takes up a lot of memory because each word or part of the input needs to be stored with the extra details of the context windows.
DeepSeekMLA, which stands for multi-head latent attention, figured out how to compress the context window, saving a lot of memory and making the model much more efficient to use.
So, I was not surprised that I saw Matthew Prince, founder and CEO of Cloudflare (NET) post this on X:
And he added:
Boom. Matthew Prince never holds back. You will see another example of that later in this article. Of course, if this efficiency is applied to the whole industry, many more LLMs could be used on the edge, where Cloudflare operates.
Another element that supports DeepSeek's claim that it used H800 chips is that DeepSeek introduced a third crucial innovation. It programmed 20 out of the 132 processing units on each H800 chip to handle the communication between the chips. More on this later.
The technology's open-source nature is also revolutionary. This means everyone can examine the model, modify it, and develop it further. This could lead to a revolution in which everyday people without the deep pockets of Big Tech can start working with the code to develop new things.
For a quite a while already, I have been saying we were still in phase 1 of the AI revolution. This may mean the start of phase 2.
The infrastructure is the most important aspect of the first phase. We've seen this in Nvidia's meteoric rise.
But the second wave is where things are built on top of the existing infrastructure. The large-language models have never been the ultimate application of all this. With the efficiency that DeepSeek adds to this, no matter if they lie about the number and type of Nvidia's GPUs, a new era seems to start.
Don't forget that the LLMs (ChatGPT, Perplexity, Claude, Grok, Llama, etc.) have never been seen as the end goal, but always as an intermediary stop on the way to AGI (Artificial General Intelligence).
If DeepSeek can do this on 50,000 H800 chips, how much more can the hyperscalers do with their much bigger supply of H100 chips and even more advanced chips like Nvidia's Blackwell?
Microsoft bought 450,000 H100 chips in 2024, Meta 350,000, Amazon about 200,000 and Google about 175,000. If they can apply DeepSeek's efficiency to their higher number of GPUs, the results may be even more breathtaking.
So, does DeepSeek introduce a new era in artificial intelligence? My best guess is yes. On Saturday, I posted an X poll about this, and a majority of voters thinks we are entering a new phase of AI.
If you like my work, consider supporting me with a paid subscription.
Censorship
There are limits to DeepSeek, though, as the model is censured. Don't ask for Winnie The Pooh (which is the nickname of the Chinese president Xi Jinping) or about the Tiananmen Square uproar. And the answer about Taiwan is also completely in line with the Chinese Communist Party.
(Made by the author)
The Implications For Nvidia & Others
Unsurprisingly, Nvidia's stock is down a lot today, along with other chip makers like Broadcom (AVGO) and energy companies that serve data centers like Vistra (VST) and NRG (NRG).
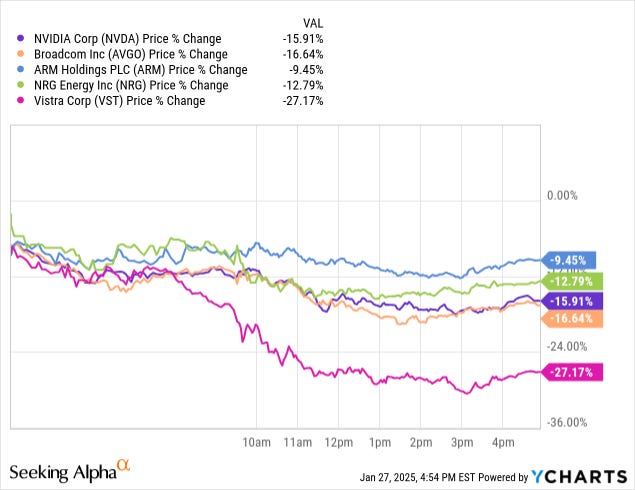
Of course, I understand the reasoning, and so will you: The increased efficiency does not look good for Nvidia.
Especially if you know that DeepSeek circumvented CUDA, which I saw as its moat up to now. CUDA stands for Compute Unified Device Architecture. Nvidia's platform allows developers to use GPUs for general-purpose computing, not just graphics. It's been fundamental for AI so far.
Earlier in this article, I wrote that DeepSeek programmed 20 out of the 132 processing units on each H800 chip to handle the communication between the chips.
You can’t do this with CUDA. Instead, the company used PTX, which is like an assembly language for Nvidia GPUs. That's probably also part of the reason why Nvidia dropped so much today. Until yesterday, everybody agreed that CUDA had such a moat that it could not be circumvented or replaced.
And yes, Matthew Prince, Cloudflare's CEO, was provocative again about this and questioned CUDA overall.
So, it feels like Nvidia is screwed, right?
Well, not so fast.
The Jevon Paradox
Besides the fact that DeepSeek is trained on Nvidia chips (be it the H800 or illegal H100s), there's also the Jevon Paradox.
This is how ChatGPT describes it (do you see the irony in using ChatGPT for this? It was intentional):
The Jevons Paradox, named after the English economist William Stanley Jevons, refers to the counterintuitive phenomenon where increases in the efficiency of resource use lead to an overall increase in the consumption of that resource, rather than a decrease.
Key Idea:
When technology improves the efficiency of resource usage (e.g., energy, raw materials), the cost of using that resource decreases. This often leads to:
Increased demand for the resource because it becomes cheaper to use.
Broader adoption of the resource in applications that were previously uneconomical.
Overall consumption rising rather than falling.
Examples:
Coal in the 19th Century: Jevons originally observed this phenomenon with coal in his book The Coal Question (1865). He noted that improvements in steam engine efficiency (which reduced coal consumption per unit of output) led to an increase in total coal consumption because coal-powered applications became more cost-effective and widespread.
Fuel-Efficient Cars: Modern fuel-efficient cars use less fuel per kilometer, reducing the cost of driving. However, people may respond by driving more or purchasing larger vehicles, potentially increasing total fuel consumption.
Lighting Efficiency: Advances in lighting, such as LED technology, dramatically reduced the energy needed for lighting. But because lighting became cheaper, it led to more usage (e.g., lighting up more areas or keeping lights on longer), which can result in higher overall energy consumption.
This is a graphic illustration of the Jevons Paradox in the context of IT services:
And this is Jevon's Paradox in the context of hybrid cars:
In the context of the Jevons Paradox, if LLMs can be made more efficient and need fewer Nvidia chips, according to Jevon's Paradox, there should be more usage through increased consumption.
But of course, this is for the long term. Over the short term, I think the market won't be soft on Nvidia. Most will see this either as Nvidia going the way of Cisco after the dotcom bubble (I'm definitely not in that camp) or just another dip in the cyclical lives of chip companies. And that could be the case, for sure. The only catalyst for the shorter term could be the earnings.
But don't forget that the hyperscalers are eying AGI, artificial general intelligence. The current state of AI is just an intermediary stop towards AGI.
With DeepSeek's efficiencies added to the models, American companies will have to adopt the newer models. DeepSeek's open-source model has the potential to undercut Big Tech's monopolies in AI.
This is a great development for Amazon (AMZN) and Apple (AAPL), who don't have their models. Meta, which is developing its own open-source LLM with Llama, can say that this open-source model shows that it's following the right approach to AGI.
The Moravec Paradox
The Moravec Paradox is another element to consider. It is the paradox named after Hans Moravec that tasks humans find easy are often very hard for computers, while tasks humans find hard are relatively easy for computers.
For example:
A toddler can walk, recognize faces, or hold a conversation with ease, but these require extremely complex programming and computing power for machines.
On the other hand, solving math problems or playing chess at a superhuman level, which many humans find difficult, is relatively straightforward for computers.
The paradox exists because activities like walking or recognizing objects rely on millions of years of evolution and subconscious processes, which are deeply rooted in our brains.
Now, what does this have to do with Nvidia and its future? Nvidia is working on the physical side of AI, which means they are trying to combine AI and robotics. A few weeks ago, the Nvidia GB10 was presented at the CES conference in Las Vegas.
This shows that Nvidia is not waiting for the market to show what it wants. It actively skates to where the puck will be in the future. That means that the company will not be the next Cisco anytime soon.
Just like the LLM models can implement and build on the innovations of DeepSeek, Nvidia can do that as well, for example, by adding more chip programming flexibility into CUDA.
So, over the long term, I am not really worried about Nvidia. Over the short term, though, anything can happen. As most of you know, the market trades on sentiment over the short term, not fundamentals.
Dropping Another Bomb Today
It seems DeepSeek is not done dropping bombs. They just launched Janus-Pro 7B (again open source) and claim it outperforms OpenAI's DALL-E 3 text-to-image generator across multiple benchmarks. After R1, I have no reasons to doubt this. It will be another boost for AI development because no matter what you think about a Chinese company doing this, nobody who knows something about this denies that this is a big leap forward. I have not been able to study this in all of its consequences, but I think the impact is somewhat similar to R1.
The End Of America As A Tech Leader?
I saw some claims that this is the end of the American leadership in tech. That's not the case. But it's a great reminder to continue to innovate and start from first principles. Up to now, the answer to the question of how to make better AI models was just throwing more money to Nvidia.
DeepSeek is a great reminder for Silicon Valley not to get complacent. After all, we should not forget that breakthrough innovations usually don't come from gigantic companies but from smaller players at the edge. And that's exactly what happened here. This is not a reason to panick, but it is a reason to continue to push for innovation.
If American companies implement DeepSeek innovations without restrictions on top-notch chips, it will propel us forward even faster.
What I Will Do With My Chip Positions
I see this as a temporary drop in the stock price of chip companies. But don't get me wrong. Temporary can mean two years, though. We could see attractive prices for some chip stocks. But I am not sure about this. The market may recognize the long-term potential as well and a company like Nvidia could see benefits from its GB10 faster because of the current developments.
All in all, this is a very exciting time to live in.
If you appreciated this article, you would do me a favor by sharing it!
In the meantime, keep growing!
Hi Kris. I read everything there was to read yesterday about this breathtaking innovation but your article is by far the most complete for me as a shareholder of Nvidia. Thx for acting so quickly and efficiently.
Best thing I’ve read on this topic yet. Great work.